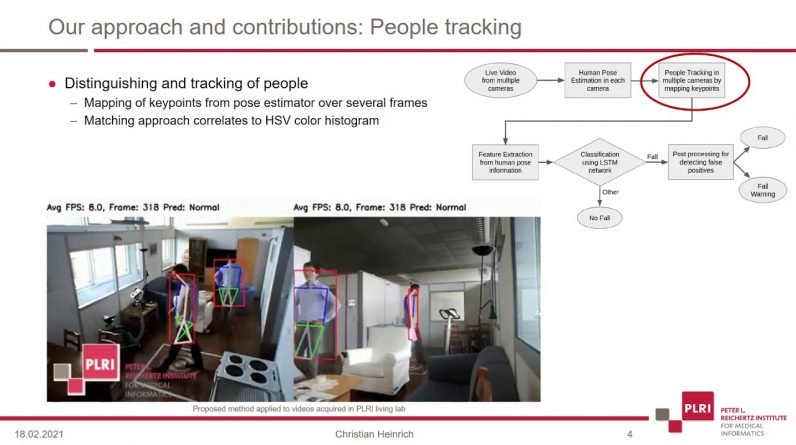
Falls occurring at home are a high risk for elderly living alone. Several sensorbased methods for detecting falls exist and – in majority – make use of wearable or ambient sensors. The field of video-based fall detection is emerging; however, restricted view of single cameras, distinguishing/tracking of persons, and high false-positive rates pose limitations. Hence, a novel approach for video-based fall detection was proposed [1]: The human pose estimation algorithm openpifpaf was augmented for fall detection by adding multi-camera and multi-person tracking support. For each person, five temporal and spatial features are extracted and processed by a long short-term memory (LSTM) network, classifying each frame as a fall or no fall event. The UP-fall detection dataset was used for evaluation, achieving a F1-score of 92.5%. Still, a trend of false-positives was observed due to imbalance as the dataset contains 36% videos showing falls; contrary to their rare occurrences in real life. We aim to improve this work by integrating it in a smart home laboratory: We will acquire a multi-camera dataset representing falls and everyday activities extending the binary to multi-class classification.
References:
Heinrich C., Koita S., Taufeeque M., Spicher N., Deserno T.M. (2021) Abstract: Multi-camera, Multi-person, and Real-time Fall Detection using Long Short Term Memory. In: Palm C., Deserno T.M., Handels H., Maier A., Maier-Hein K., Tolxdorff T. (eds) Bildverarbeitung für die Medizin 2021. Informatik aktuell. Springer Vieweg, Wiesbaden. https://doi.org/10.1007/978-3-658-33198-6_29